Downtime can cripple productivity, and that is why predictive maintenance plays a huge role in keeping machines healthy and factories efficient. Still, many businesses find their efforts fall short, not because predictive maintenance does not work, but because they make avoidable errors along the way. No matter how solid your predictive maintenance strategy seems, overlooking common pitfalls can undo your progress and lead to costly surprises.
In this article, we explore the five common predictive maintenance mistakes to avoid so you can spot trouble before it starts and keep your operations running smoothly.
In this article, we explore the five common predictive maintenance mistakes to avoid so you can spot trouble before it starts and keep your operations running smoothly.
We will uncover
- Why Predictive Maintenance Fails Without the Right Strategy
- 5 Common Predictive Maintenance Mistakes to Avoid
- Mistake #1 – Relying on Incomplete or Poor-Quality Data
- Mistake #2 – Ignoring Small Anomalies and Early Warning Signs
- Mistake #3 – Failing to Align Maintenance Goals with Business Objectives
- Mistake #4 – Not Training Staff to Use Predictive Maintenance Tools
- Mistake #5 – Overlooking the Importance of Continuous Improvement
3. Making Predictive Maintenance Work for You Using the Right Strategies
Why Predictive Maintenance Fails Without the Right Strategy
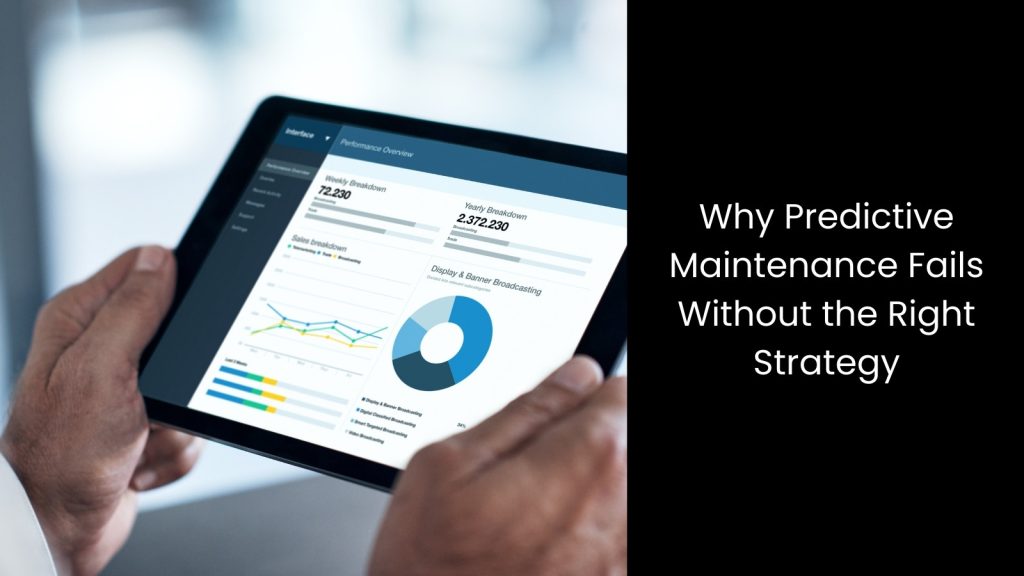
- Predictive maintenance uses real-time data and smart technology to spot signs of wear or damage in machines before they break down, helping workers fix problems early and avoid unexpected shutdowns. It brings huge benefits like reducing downtime, lowering repair costs, and keeping machines running longer.
- However, without a clear predictive maintenance strategy, it usually falls apart fast.
- It is visible that many companies jump in without proper maintenance planning, and that is where things go wrong. They usually do not set clear goals, ignore how different systems work together, or rely on random data that does not tell the full story.
- These gaps confuse the team, delay actions, and make the system useless.
- When you do not plan well, you end up fixing machines too late or too often, which still causes equipment failure and waste. A strong strategy maps out exactly what data to track, when to inspect parts, and how to respond when something seems off.
- It helps the workers stay focused, avoid guesswork, and make smarter decisions fast.
5 Common Predictive Maintenance Mistakes to Avoid
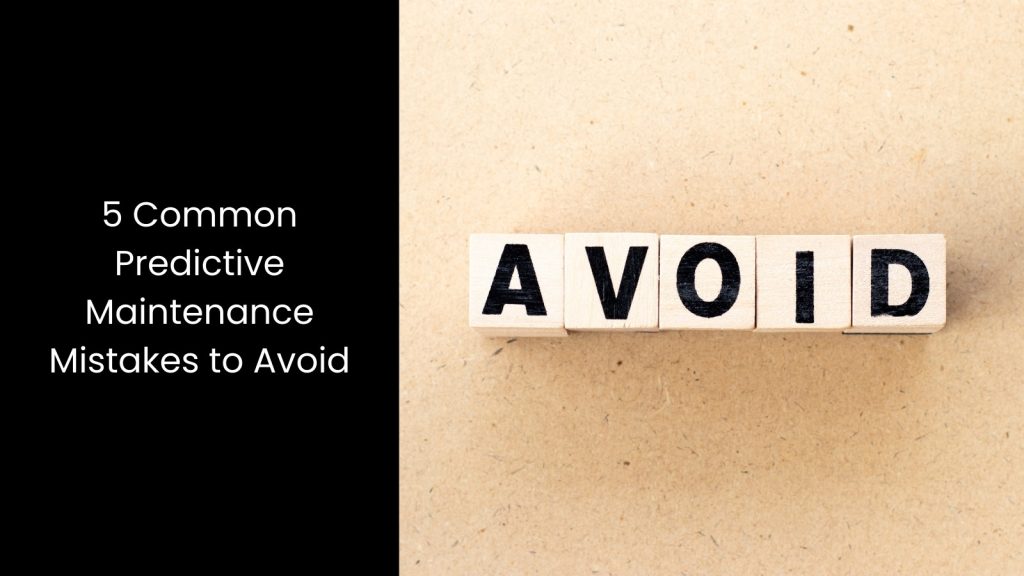
Mistake #1 – Relying on Incomplete or Poor-Quality Data
Many companies step into predictive maintenance, thinking the system will magically work, but they forget that everything depends on one thing, which is good data.
Without clean and complete data, nothing makes sense, and the whole setup starts to fall apart.
When workers rely on inaccurate readings, messy records, or scattered information stuck in different tools, they cannot trust the alerts or decisions that come next. The data analysis goes off track, and maintenance teams end up chasing the wrong problems or ignoring real ones.
In some cases, the system may completely miss early warning signs during machine condition monitoring, which means small issues can turn into costly breakdowns. Even worse, poor sensor data quality can trigger false alarms that waste time and drain resources.
So, instead of running smoothly, your machines get stuck in a loop of confusion and chaos. To fix this, always test sensors regularly and replace faulty ones before they mess with your numbers.
You can connect all your machines to a central platform that stores and syncs the data in real-time, so you never deal with gaps or duplicates. Also, label every data point clearly—things like time, location, and equipment ID, because that makes reports easier to read and act on.
Finally, let your team know what to look for during inspections and set up alerts that make sense for your machines. When you clean up the data and keep it consistent, your system can actually detect real problems early and help your crew respond with confidence.
Without clean and complete data, nothing makes sense, and the whole setup starts to fall apart.
When workers rely on inaccurate readings, messy records, or scattered information stuck in different tools, they cannot trust the alerts or decisions that come next. The data analysis goes off track, and maintenance teams end up chasing the wrong problems or ignoring real ones.
In some cases, the system may completely miss early warning signs during machine condition monitoring, which means small issues can turn into costly breakdowns. Even worse, poor sensor data quality can trigger false alarms that waste time and drain resources.
So, instead of running smoothly, your machines get stuck in a loop of confusion and chaos. To fix this, always test sensors regularly and replace faulty ones before they mess with your numbers.
You can connect all your machines to a central platform that stores and syncs the data in real-time, so you never deal with gaps or duplicates. Also, label every data point clearly—things like time, location, and equipment ID, because that makes reports easier to read and act on.
Finally, let your team know what to look for during inspections and set up alerts that make sense for your machines. When you clean up the data and keep it consistent, your system can actually detect real problems early and help your crew respond with confidence.
Mistake #2 – Ignoring Small Anomalies and Early Warning Signs
Small quirks whisper louder truths than a blaring siren, yet many crews shrug them off and race ahead, then panic when production grinds to a halt.
A low rattle, a tiny oil temperature bump, or one odd vibration spike looks harmless at first, but these early warning signs shout that gears grind, seals crack, or circuits sweat. When teams dismiss the hints, heat builds, wear spreads, and hidden fatigue snowballs into full-scale machine failure at the worst moment.
If you opt for smart planning, you should stay curious; the workers tune dashboards to fire predictive alerts. The instant readings drift from normal, and they trust those nudges enough to act fast. They walk to the line, listen, touch, and pull samples, while maintenance managers reshuffle tasks so technicians tighten bolts or swap bearings long before the schedule says so.
Proactive moves cost minutes and a few spare parts, whereas a seized shaft devours days, overtime, and customer goodwill.
A low rattle, a tiny oil temperature bump, or one odd vibration spike looks harmless at first, but these early warning signs shout that gears grind, seals crack, or circuits sweat. When teams dismiss the hints, heat builds, wear spreads, and hidden fatigue snowballs into full-scale machine failure at the worst moment.
If you opt for smart planning, you should stay curious; the workers tune dashboards to fire predictive alerts. The instant readings drift from normal, and they trust those nudges enough to act fast. They walk to the line, listen, touch, and pull samples, while maintenance managers reshuffle tasks so technicians tighten bolts or swap bearings long before the schedule says so.
Proactive moves cost minutes and a few spare parts, whereas a seized shaft devours days, overtime, and customer goodwill.
Mistake #3 – Failing to Align Maintenance Goals with Business Objectives
Many workers build a strong predictive maintenance strategy, but they forget to match it with what the business actually needs. That is where everything starts to fall apart.
If the maintenance crew focuses only on fixing machines quickly while the company wants to cut energy use or improve delivery times, then people pull in different directions and waste time, money, and effort. This kind of poor business alignment leads to confusion, delays, and missed goals.
You might replace parts too early, monitor the wrong machines, or run reports that no one uses. To stop this, sit down with both operations and leadership before setting your plan. Talk about which machines impact the most profit, which breakdowns hurt your schedule, and what success looks like.
Then, shape your maintenance tasks around those goals. Use asset performance management tools to track uptime, repair costs, and energy use, and link those numbers to business KPIs like on-time delivery or product quality. Also, update the plan every few months so it keeps up with shifting targets or market changes.
Teams that stay flexible and talk often can spot what works and what does not, then adjust fast. That saves time and keeps the maintenance work meaningful, not just busy.
If the maintenance crew focuses only on fixing machines quickly while the company wants to cut energy use or improve delivery times, then people pull in different directions and waste time, money, and effort. This kind of poor business alignment leads to confusion, delays, and missed goals.
You might replace parts too early, monitor the wrong machines, or run reports that no one uses. To stop this, sit down with both operations and leadership before setting your plan. Talk about which machines impact the most profit, which breakdowns hurt your schedule, and what success looks like.
Then, shape your maintenance tasks around those goals. Use asset performance management tools to track uptime, repair costs, and energy use, and link those numbers to business KPIs like on-time delivery or product quality. Also, update the plan every few months so it keeps up with shifting targets or market changes.
Teams that stay flexible and talk often can spot what works and what does not, then adjust fast. That saves time and keeps the maintenance work meaningful, not just busy.
Mistake #4 – Not Training Staff to Use Predictive Maintenance Tools
Companies invest their money into smart sensors and software, but if no one knows how to use them, the tools just gather dust while breakdowns keep happening.
That is the trap many companies fall into. They skip technical training, hand over the system, and expect results overnight. Predictive systems need more than flashy dashboards; they need people who understand what the data means and how to act on it.
If they do not have proper guidance, workers either ignore alerts or panic when they do not know what a reading shows. The team starts guessing instead of planning, and the whole maintenance planning process turns into a guessing game. Machines stay down longer, repairs get delayed, and people lose trust in the technology.
To fix this, start with hands-on lessons where staff actually use the predictive maintenance tools on real machines, not just sit through boring slides. Encourage questions, give them time to explore the system, and break down the features into bite-sized steps.
Don’t stop after one session either; build a habit of ongoing support, monthly refreshers, and quick updates when tools change.
That is the trap many companies fall into. They skip technical training, hand over the system, and expect results overnight. Predictive systems need more than flashy dashboards; they need people who understand what the data means and how to act on it.
If they do not have proper guidance, workers either ignore alerts or panic when they do not know what a reading shows. The team starts guessing instead of planning, and the whole maintenance planning process turns into a guessing game. Machines stay down longer, repairs get delayed, and people lose trust in the technology.
To fix this, start with hands-on lessons where staff actually use the predictive maintenance tools on real machines, not just sit through boring slides. Encourage questions, give them time to explore the system, and break down the features into bite-sized steps.
Don’t stop after one session either; build a habit of ongoing support, monthly refreshers, and quick updates when tools change.
Mistake #5 – Overlooking the Importance of Continuous Improvement
Many teams treat predictive maintenance like a one-time setup, thinking that once the system runs, it will just work perfectly forever. This mindset causes big problems because predictive maintenance is not a ‘set and forget’ kind of deal.
Machines age, conditions change, and new data keeps coming in, so your approach needs constant attention. Skipping continuous improvement means your system grows outdated fast, misses new failure signs, or wastes resources on outdated checks.
To keep things running smoothly, maintenance managers must regularly review their plans, check what is working, and adjust where needed. This ongoing process of maintenance optimisation ensures the strategy matches real-world conditions and business needs. Setting up a strong feedback loop between operators, technicians, and analysts makes a huge difference here.
When teams share what they see in the field and compare it with system predictions, they spot gaps, fix false alarms, and fine-tune alert thresholds.
That constant back-and-forth helps the system learn and improve its accuracy over time.
Machines age, conditions change, and new data keeps coming in, so your approach needs constant attention. Skipping continuous improvement means your system grows outdated fast, misses new failure signs, or wastes resources on outdated checks.
To keep things running smoothly, maintenance managers must regularly review their plans, check what is working, and adjust where needed. This ongoing process of maintenance optimisation ensures the strategy matches real-world conditions and business needs. Setting up a strong feedback loop between operators, technicians, and analysts makes a huge difference here.
When teams share what they see in the field and compare it with system predictions, they spot gaps, fix false alarms, and fine-tune alert thresholds.
That constant back-and-forth helps the system learn and improve its accuracy over time.
Making Predictive Maintenance Work for You Using the Right Strategies
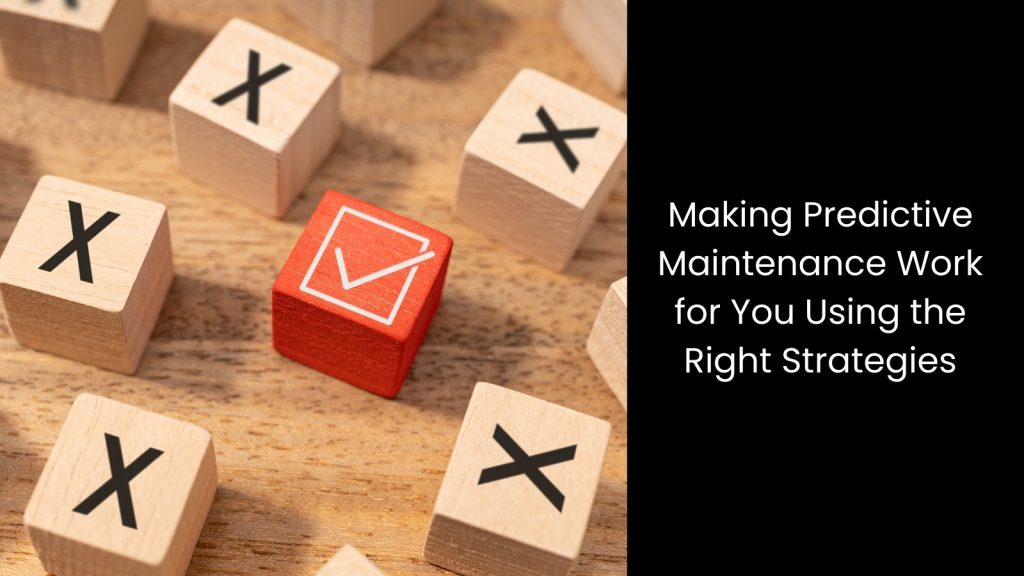
If you want your predictive maintenance to pay off, you need more than sensors. You need a solid predictive maintenance strategy, partnering with a reputable software provider. Nail your data, match maintenance with business goals, and keep refining your process. That is how you move from firefighting to true asset performance management success.