Machine learning (ML) is a popular aspect of artificial intelligence (AI). The integration of such technologies provides data analytical capabilities, specifically offering futuristic insights in terms of informing companies of the changing consumer and market changes or forewarning asset anomalies that could lead machines to break down and affect factory production. All this, however, depends on the wide accumulation of data through multiple machine-learning models. This has led to several unethical ways of data gathering, breaching data privacy, and risking the security of internet users. A more advanced stage of machine learning that has recently been developed is federated learning.
It is essentially a more modern way of training AI models to unlock information when feeding on new AI applications. For a long time, companies integrating AI have been encouraging software to integrate all data in their company into one central location because it is the most reliable way of receiving more accurate data. However, federated learning focuses on data decentralisation which processes data at its very source. It distributes the training of models across user devices, making it possible to take advantage of machine learning while significantly reducing the need to collect large amounts of user data. This article will provide an overview of what federated learning is.
How New Is Federated Learning?
While federated learning is a far more modern concept than other learning technologies, it is not a model that emerged recently. However, federated learning received more popularity against the backdrop of many countries taking action against the distrusting nature of the world wide web, as it was becoming clear that personal data was being shared and used by unknown parties in the virtual world. Hence, in 2016, Google introduced federated learning in an attempt to provide a solution for data protection or privacy concerns. The immediate context that led to this was the famous Cambridge Analytica scandal. The scandal was one of the key global incidents demonstrating the danger of sharing personal information online. The incident also led to the sensitive topic of users on the internet being tracked by unknown strangers without their consent. The lack of consent in an online platform has led more people to be careful using the internet. Certain countries have taken a clear stance in this regard by introducing laws. Hence, the European Union countries implemented the GDPR law, which required a business or a person to have a mechanism in place to ensure that they received a person’s consent to use their data. Other countries or states such as California, Brazil, Argentina, and Canada also passed relevant laws on data protection.
What Does It Mean To Have A Centralised Machine Learning Model?
Centralised ML refers to the creation of an algorithm based on training data. This includes sample data that allows companies to identify patterns and trends. The machine thereafter uses the algorithms developed to learn such patterns and identify them in enormous data pools. Therefore, the traditional form of ML is centralised, whereby all data is accumulated in one location. The best example is demonstrated by Google maps, which provides the fastest possible route or alternate routes depending on the user’s location and destination. Google does this by accessing the data the server has collected through multiple vehicles that used this same route for travelling. In other words, Google looks at how many people have taken a similar route from their central data database and transfers the relevant information to you. As almost anyone today has used Google Maps, the importance of such an app is appreciated. However, despite the flexibility it provides to the consumer, the fact that central data is stored leads to a violation of user privacy and risks personal data. Unknowingly most companies record user data in their system, and on this basis, consumers enjoy more relevant posts on their socials. In addition to such issues, SMEs, in particular, may find it hard to gain accurate results due to the low amount of data. Finding ways to accumulate ample data ethically to gain better insights has been a problem such companies are facing.
How Does Federated Learning Work?
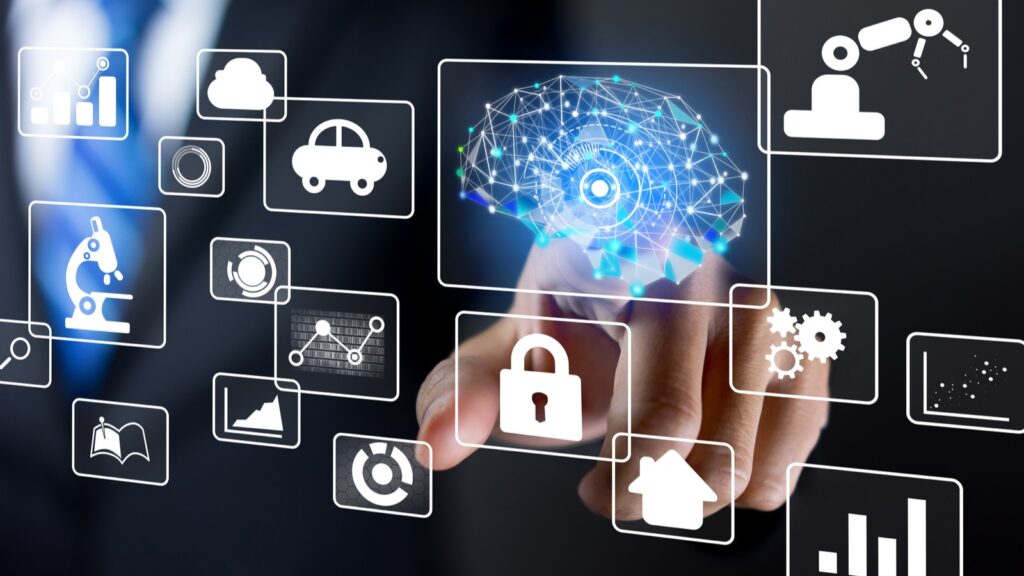
As federated learning focuses on ethically extracting data from their sources, the main focus of this mode of learning is to train AI models. Unlike ML, which had to store data from multiple devices on a cloud, federated learning trains ML models on user data without needing to send them to the cloud. However, to do this, federating learning first starts with a base ML model, which is connected to the cloud and has either been trained or not trained on public data. This makes federated learning a decentralised form of machine learning. The second stage of federated learning includes the voluntary participation of user devices to train the model only to use relevant user data for the model application. In the third stage, the devices download the base ML and train it to work on the local data of the device. After the training stage, the training model is removed as a fourth step, whereby they can independently encode the statistical patterns of data in numerical parameters without training data. As a result, once the training model is removed, the device does not contain raw user data. This leads to creating a system that encrypts user-sensitive data that is not in a centralised cloud server. This process is known as the Secure Aggregation Principle. It allows the server to secure and combine the encrypted results and decrypts only the aggregated results.
In short, a device will download the existing ML model, improve it by learning from the data in the device in question, and summarise the changes as an update. The update is sent to the encrypted cloud and averaged with other user updates to improve the shared model. A famous example is the use of the Google keyboard on android devices. Also referred to as a Gboard, a person initially uses the typical and general keyboard provided by Google, which subsequently improves and offers better suggestions to the user upon the information stored in their phone. It will consider consumer user patterns and use such information to improve the app.
But Does Federated Learning Really Eliminate Privacy Issues?
In terms of security, the ideal model that, on an ethical level, is proper and that consumers will respect is federating learning. However, federated learning does not completely eliminate all privacy issues. For instance, since the federated learning model depends on the ML model in its creation, data on model updates in the training process can reveal sensitive information, either to a third party or to the central server. However, there are protocols that can be implemented to curtail this. This includes using a secure multiparty computation (SMC) or differential privacy. SMC distributes computation amongst multiple parties, although each individual party cannot see one other’s data. Hence, SMC fully embraces the concept of additive secret sharing, which establishes a proper division of secrets as data is not transferred outside their internal firewall. This is a famous cryptographic protocol used in the world today to combat financial fraud. Differential privacy refers to an approach similar to SMC that provides information without divulging the personal identification of the other. To do this, a minimum distraction is required. The piece of information that acts as a distraction is large enough to protect privacy while, at the same time, it is also limited since useful data can still be provided to analysts. In other words, differential privacy injects noise into a dataset allowing data experts to execute possible statistical analyses without identifying any personal information. A few examples of this protocol being implemented in practice include Apple, which draws usage insights on a user’s time spent using their Apple devices, while Facebook uses it to draw behavioural data for target advertising campaigns without infringing national privacy laws.
Implementing SMC or differential privacy protocol measures, however, may not yet be the perfect solution. They may restrict and hinder model performance significantly. It may also pose a problem in preserving deep learning. For instance, in SMC, computational overhead leads to the run time of the computation being significantly slow. It also leads to higher communication costs compared to traditional plaintext computing. In differential privacy, there is no actual guarantee that a user’s secret will remain a secret, as the system may not be able to make a distinction between casual and private data. Since differential privacy moreover focuses on protecting specific information that is viewed as private, if the information is considered general, despite it being an actual secret, it will not protect it.
What Are the Other Challenges of Federated Learning Poses?
In order for federated learning to work, it requires a global statistical model from data stored in multiple devices. It is, in practice, hard to find one single statistical model. Additionally, although federated learning is most suited for companies that use data from a large number of devices, the larger it is, the slower the network gets during local computation. It is also a more costly alternative. The biggest challenge, which is contrary to the idea that federated learning is a solution against data security and privacy, is that federated learning does not completely eliminate all privacy concerns, as explained above. This is especially true during the sharing model update stage, which provides gradient information instead of raw data. Hence, model updates throughout the training process may result in sensitive information being leaked to a third party or to the central server rather than remaining in the local server.