Imagine a vast ocean of information swirling in unstructured text- emails, reports, social media posts, research papers, and more. This flood of data can offer incredible insights for Australian businesses and research sectors if only there were a way to make sense of it. This is where ‘Textual Data Mining’ comes in, transforming unorganised text into structured, meaningful insights. By tapping into these insights, Australian organisations can uncover trends, predict market shifts, and enhance decision-making.
This article covers everything about ‘Textual Data Mining’ and how it unlocks hidden value.
This article covers everything about ‘Textual Data Mining’ and how it unlocks hidden value.
Understanding Textual Data Mining
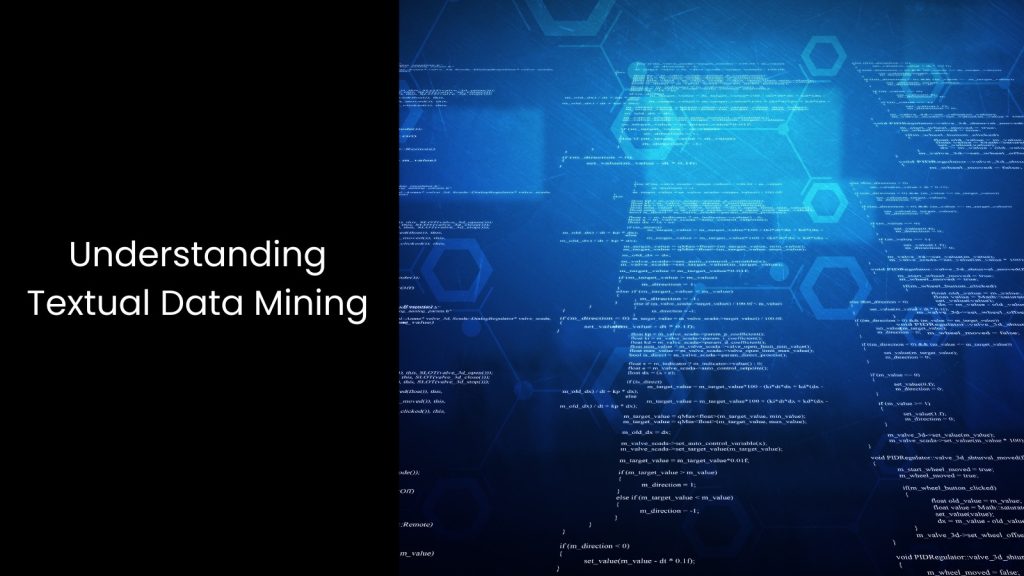
- Textual data mining, also known as text mining, enables businesses to extract valuable insights by transforming raw text into structured formats. Through this process, companies can reveal hidden patterns, relationships, and trends in vast volumes of text.
- The training phase of a textual data mining process involves applying sophisticated analytical techniques, such as text analysis and textual analytics, to capture key concepts and discover connections. Advanced algorithms, including Naive Bayes, Support Vector Machines (SVM), and deep learning models, play crucial roles in analysing and categorising unstructured data like social media posts, product reviews, and other text-rich content.
- Now let us cover its mechanism. Textual data comes in three primary forms: structured, semi-structured, and unstructured. Structured data, organised in rows and columns, makes analysis easier for machine learning. Examples of structured data include names, addresses, and phone numbers, where information follows a predictable pattern. On the other hand, unstructured data lacks a predefined format and might consist of text sources like online reviews or even multimedia files, which cannot be easily organised in a standard database format. Semi-structured data, like JSON, XML, and HTML, sits in between, and it has some format but lacks the rigid structure necessary for relational databases.
- According to the experts, about 80% of the world’s data is unstructured. This is why textual data mining becomes essential for organisations to make sense of vast information pools.
Is Text Mining and Text Analytics Same?
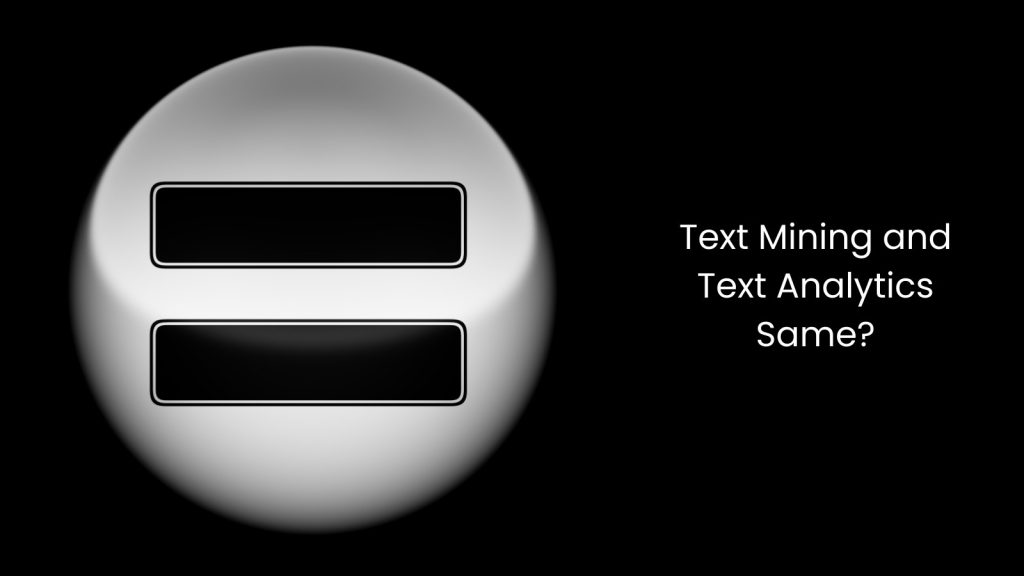
It is not a secret that many get confused here.
While people usually use text analysis and textual data mining interchangeably, they come with slightly different meanings.
As you can see, text mining involves discovering patterns and trends in unstructured text data by using machine learning, statistical methods, and language processing techniques. This process transforms raw data into a structured format, making it easier to analyse.
Text analytics, on the other hand, builds upon this foundation by applying quantitative methods to these structured results, aiming to gain deeper insights. Data visualisation tools come into play in text analytics, allowing organisations to present findings to a broader audience effectively.
As it is evident, both text mining and text analytics work together to help businesses unlock insights hidden within vast amounts of text data, but text mining emphasises the structuring phase, while text analytics focuses on analysing and interpreting structured information for practical, data-driven decisions.
While people usually use text analysis and textual data mining interchangeably, they come with slightly different meanings.
As you can see, text mining involves discovering patterns and trends in unstructured text data by using machine learning, statistical methods, and language processing techniques. This process transforms raw data into a structured format, making it easier to analyse.
Text analytics, on the other hand, builds upon this foundation by applying quantitative methods to these structured results, aiming to gain deeper insights. Data visualisation tools come into play in text analytics, allowing organisations to present findings to a broader audience effectively.
As it is evident, both text mining and text analytics work together to help businesses unlock insights hidden within vast amounts of text data, but text mining emphasises the structuring phase, while text analytics focuses on analysing and interpreting structured information for practical, data-driven decisions.
Top Applications of Textual Data Mining
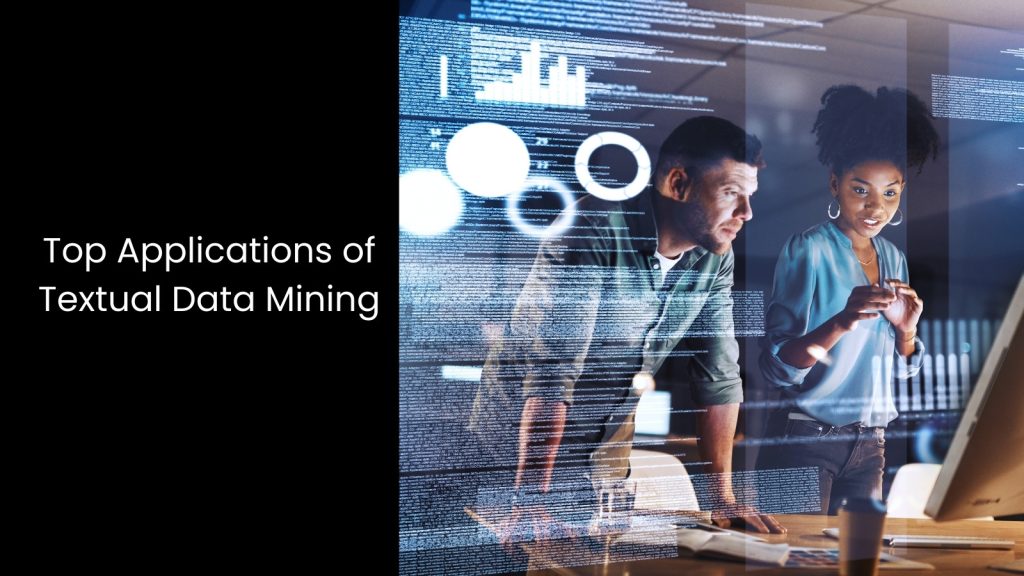
Maintenance Initiatives
You know that Australian authorities face a constant challenge in managing the maintenance of massive infrastructure projects effectively, especially given the overwhelming amount of data available.
Text mining and text analytics provide valuable tools for tackling this issue, using textual data mining to organise and interpret vast information about equipment and machinery performance. As it can analyse data from inspection reports, incident logs, and maintenance records, these tools help identify patterns that indicate potential problems or necessary repairs.
Over time, text mining becomes even more powerful over time, as it automatically uncovers connections between recurring issues and specific maintenance practices, aiding in proactive and reactive decision-making.
Through text mining, maintenance teams can monitor equipment operation in detail, enabling a comprehensive view of how different components perform under various conditions. Text analytics then builds on this by applying structured analysis to uncover the root causes of frequent breakdowns or issues, pinpointing exactly why and where failures occur. These insights streamline problem-solving and reduce downtime, as technicians can quickly identify what actions to take based on established patterns and previous cases. This automated approach not only saves time but also cuts costs by preventing minor issues from escalating into major repairs.
Text mining and text analytics provide valuable tools for tackling this issue, using textual data mining to organise and interpret vast information about equipment and machinery performance. As it can analyse data from inspection reports, incident logs, and maintenance records, these tools help identify patterns that indicate potential problems or necessary repairs.
Over time, text mining becomes even more powerful over time, as it automatically uncovers connections between recurring issues and specific maintenance practices, aiding in proactive and reactive decision-making.
Through text mining, maintenance teams can monitor equipment operation in detail, enabling a comprehensive view of how different components perform under various conditions. Text analytics then builds on this by applying structured analysis to uncover the root causes of frequent breakdowns or issues, pinpointing exactly why and where failures occur. These insights streamline problem-solving and reduce downtime, as technicians can quickly identify what actions to take based on established patterns and previous cases. This automated approach not only saves time but also cuts costs by preventing minor issues from escalating into major repairs.
Filtering Spams
Did you know Australian systems face thousands of hacking attempts and spam attacks annually?
Many spam emails act as gateways for hackers, slipping malware into user systems to compromise data security. Textual data mining, paired with text analytics, offers a strong defence against this by identifying and filtering out spam before it reaches users’ inboxes.
As it is possible to scan and analyse text patterns in emails, these techniques detect the markers of spam messages, such as suspicious keywords, abnormal sender information, and unusual message formats. They then classify potentially harmful emails and prevent them from landing in inboxes, boosting both security and user experience.
In the spam-filtering process, text mining breaks down the content of emails to identify repetitive spam signals across different messages, training algorithms to flag harmful patterns. Textual data mining further strengthens this by transforming unstructured text from emails into structured data, which makes it easier to apply machine learning and statistical models.
As a result, systems can more accurately separate genuine emails from spam, reducing the number of suspicious messages slipping through. Meanwhile, text analytics dives deeper into the structured data, enhancing spam detection accuracy by continuously updating filters based on new spam trends and cyber-attack methods. This dynamic learning ensures that the system stays resilient against evolving cyber threats and keeps users protected.
Many spam emails act as gateways for hackers, slipping malware into user systems to compromise data security. Textual data mining, paired with text analytics, offers a strong defence against this by identifying and filtering out spam before it reaches users’ inboxes.
As it is possible to scan and analyse text patterns in emails, these techniques detect the markers of spam messages, such as suspicious keywords, abnormal sender information, and unusual message formats. They then classify potentially harmful emails and prevent them from landing in inboxes, boosting both security and user experience.
In the spam-filtering process, text mining breaks down the content of emails to identify repetitive spam signals across different messages, training algorithms to flag harmful patterns. Textual data mining further strengthens this by transforming unstructured text from emails into structured data, which makes it easier to apply machine learning and statistical models.
As a result, systems can more accurately separate genuine emails from spam, reducing the number of suspicious messages slipping through. Meanwhile, text analytics dives deeper into the structured data, enhancing spam detection accuracy by continuously updating filters based on new spam trends and cyber-attack methods. This dynamic learning ensures that the system stays resilient against evolving cyber threats and keeps users protected.
Healthcare Field
In healthcare, textual data mining coupled with text analysis has become an essential tool for medical researchers to handle the vast amount of information available in medical literature.
Sorting through countless studies and research papers manually takes a lot of time and resources, which slows down progress in critical areas of medicine. Textual data mining automates this process, scanning through extensive collections of medical documents to extract meaningful insights and relevant data. It groups similar information together, helping researchers identify patterns, trends, and relationships in complex biomedical data.
In another way, text mining allows healthcare professionals to locate significant findings faster, like linking symptoms to diseases or assessing the effectiveness of treatments across different patient demographics. This technology also speeds up the discovery process by eliminating redundant steps and focusing researchers’ efforts on valuable data rather than exhaustive reading.
Sorting through countless studies and research papers manually takes a lot of time and resources, which slows down progress in critical areas of medicine. Textual data mining automates this process, scanning through extensive collections of medical documents to extract meaningful insights and relevant data. It groups similar information together, helping researchers identify patterns, trends, and relationships in complex biomedical data.
In another way, text mining allows healthcare professionals to locate significant findings faster, like linking symptoms to diseases or assessing the effectiveness of treatments across different patient demographics. This technology also speeds up the discovery process by eliminating redundant steps and focusing researchers’ efforts on valuable data rather than exhaustive reading.
Customer Service
The latter plays a decisive role in enhancing customer service by analysing feedback across multiple channels. Companies collect input from chatbots, customer surveys, NPS scores, online reviews, support tickets, and social media profiles, gaining a wide-ranging view of customer experiences when enriched with textual data mining capabilities. When using text analytics, businesses dig into this feedback to identify the most pressing issues, uncovering customer pain points and areas for improvement.
Sentiment analysis, a key part of text mining, helps companies gauge customer emotions, allowing them to spot negative trends or dissatisfaction in real-time.
With this data, companies can prioritise urgent problems, providing faster responses and addressing concerns before they escalate. Text mining also helps teams understand patterns in customer feedback so they can focus on recurring issues that impact satisfaction levels.
Sentiment analysis, a key part of text mining, helps companies gauge customer emotions, allowing them to spot negative trends or dissatisfaction in real-time.
With this data, companies can prioritise urgent problems, providing faster responses and addressing concerns before they escalate. Text mining also helps teams understand patterns in customer feedback so they can focus on recurring issues that impact satisfaction levels.
Structuring Data Accurately via Textual Data Mining Techniques Embedded Solutions
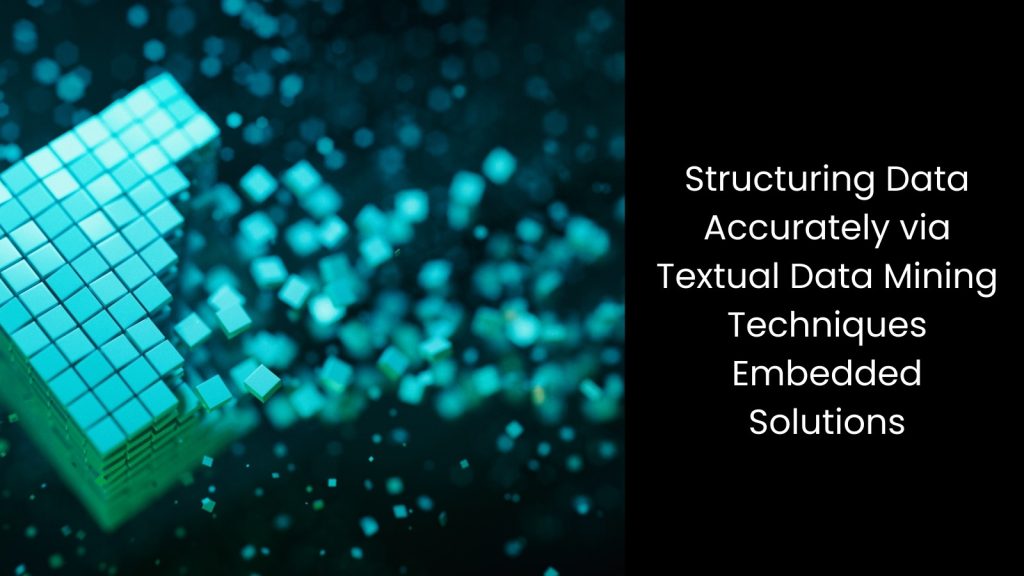
Think about what accurately structured data can do for your organisation in Australia. If you can integrate textual data mining techniques, you do not just manage information but unlock possibilities more and more. The best thing you can do is purchase a software solution that has textual data mining capabilities embedded. With the right partner, you can keep away a safe distance from misinterpreted data when it comes to your maintenance activities.